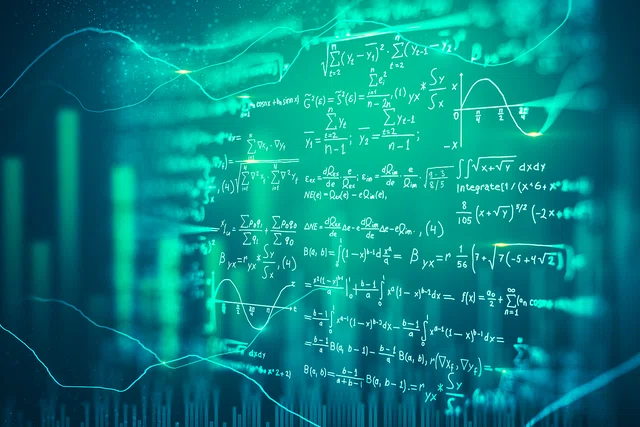

Bob Tisdale—Former CEO, Pembridge Insurance
Since retiring a year ago last August, I’ve had an opportunity to review many of the trade publications I collected during my 40-year career with Pembridge. Some of these date back to the 1970s and serve as a time capsule, preserving the challenges and concerns I’ve watched the industry confront over the decades.
THE TIMELESS CHALLENGE
One of the main recurring themes has been creating pricing adequacy to avoid insurance cycle fluctuation. Using property as an example, we know pricing is based on COPE: Construction, Occupancy, Protection and Exposure. Through data and historical experience, we know prices are reduced or increased depending on the superiority of the construction, the type of occupancy, the protection provided and what the building’s exposed to. Data gathered over the years was used to determine the amount of credit or debit to apply to the rates. Other data like claims experience and financial status evolved and became accepted measures of risk.
In automobile insurance, data gathered over 100+ years helped underwriters determine pricing adequacy. Each of these defining factors was developed as the data gained credibility. Loss history, traffic violations, location and type of vehicle are all data points that evolved as underwriters gained more experience within the class. In recent years, the availability of data has increased dramatically. Combined with superior analytics and computing, today’s algorithms and pricing methods have disrupted the historical approach to pricing. The complexity of these factors has made it much more difficult for the average person to explain pricing changes to the consumer. The so-called black box calculations have to many become so complicated they’re totally unexplainable.
I wonder if the industry faced the same dilemmas when the first credits emerged for changing technologies like sprinkler systems and burglar alarms. Perhaps these changes were easier to explain until they became accepted norms of underwriting and pricing. I know from personal experience we had a difficult time convincing the jewelers we insured in the early 80s of the merits of alarm systems and video cameras. They eventually became commonplace, but not without a lot of consternation.
THE FAMILIAR NEW
Today’s black box is no different than prior era pricing factor usage. Yes, it’s more complex, but it still does the same thing—establishes the price of the risk. The difference seems to be that the data and factors used today are more multifaceted, but still require someone to create and test their validity.
The power of today’s data analytics and computing ability allows pricing models to be much more granular than broader based historical factors. This permits pricing models to be more refined, more accurately assessing a particular risk. In historical risk pools, the best risks subsidized the worse ones due to the inability to accurately define each one. In each risk pool there’s a need to have adequate premium to pay the losses of that group of risks. Today’s difference is that each contributor to the pool will pay a premium more accurately reflective of their amount of risk. This is and has been a fundamental pricing shift especially in lines of business that aren’t heavily regulated. I recently became aware of new technologies and analytics for commercial automobile risks. This technology uses artificial intelligence and machine learning to assess risk. It’s not rules-based as are most, if not all, telematics on the market today. Instead, this technology consistently analyzes what the driver’s doing relative to what a driver should do. And when the driver deviates from this accepted behaviour, it’s flagged as an abnormal event. A video’s created to analyze what the driver did that deviated from the norm—once reviewed, a critical coaching moment occurs to help the driver correct the deviated behaviour. This is a new and more accurate approach in defining risk and ultimately creating a pricing model solely based on the behaviour of a particular driver. These drivers’ scores are combined in order to price the fleet. Eliminate the drivers with the worst scores and the experience of the fleet gets better, lowering the overall price. It’s only a matter of time until our industry broadly adopts this more modernized approach to risk assessment and pricing.
THE INEVITABLE SOLUTION
While some may use the complexity of today’s pricing models to avoid discussion with consumers, I suggest we need to embrace it. We must recognize that the data analytics and algorithms used today are an expansion of the data used in the past. They’re part of the technological advancements we’re seeing in all products, like how the modern computing power in today’s automobile dwarfs the mechanical components it replaced. This technology advancement, while much more complex, has made vehicles much more efficient and reliable—even if you can’t fix them yourself in your backyard!
The evolution of data usage and its growing complexity still hasn’t solved the insurance cycle challenge. Despite the pricing methods of a particular time, the industry still oscillates between soft and hard markets. Emerging technologies and the availability of more and more data analysis suggests to me the industry is on the right track. Yes, the so called black box is difficult to explain, but if it means more consistent underwriting results and fewer price swings, we, and more importantly our customers, will be better off.